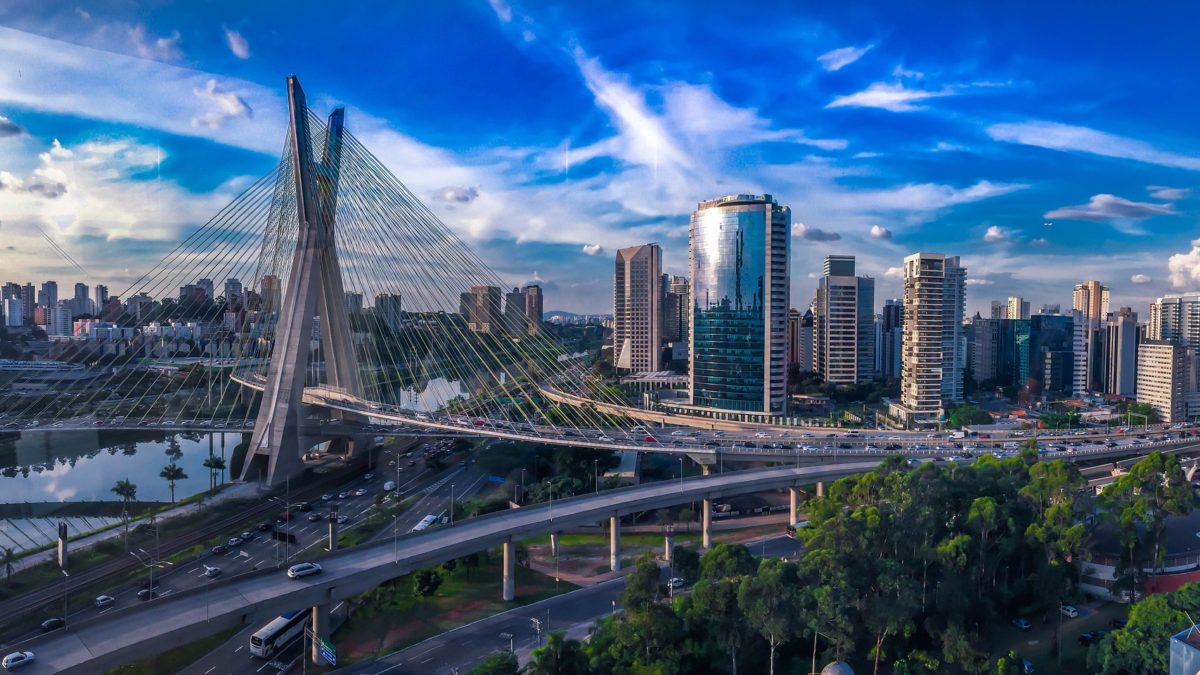
AI Driven Construction Asset Management for Operators and Maintenance Contractors – Predicting Construction Asset Conditions
This is the second article in our series on AI Driven Construction, where will explore how AI can drive construction asset management for clients, operators and maintenance contractors. You can read our first article on AI Driven Construction Management here.
Let’s jump right into it. Asset management in the engineering and construction sector is hard, whether this is the up-keeping of structures such as buildings, tunnels or roads or ensuring uptime of equipment and machinery. While there is a great variety in types of asset management, they all have one thing in common, they are labour intensive and require continuous monitoring to ensure an asset’s lifetime value is maximised.
If you have a portfolio of construction assets you need to maintain, it’s critical that you maintain an accurate overview of the condition of each asset. While our example below is focused on a portfolio of bridges across the state of New York, assets can be anything. Whether you are an engineering & construction firm, railroad operator, water utilities company or a facilities manager, you have assets that you need to maintain.
The question we will offer an answer to in this case study is, how to maintain construction asset more efficiently. More specifically we will look at how AI can be used to inspect a portfolio of 17,439 bridges across the state of New York. We are using a publicly available data set, which is available via New York State Open Data.
(Note: we are a London based firm but have found NYS makes better data available publicly than the UK Open Data portal, hence why we are using US examples)
Construction Asset Management
Before we start, think about why AI could be useful in construction asset management. New York State has a population of 19 million people is in total 87k sqm² which is roughly 1/3 of the UK’s population and over 1/2 of the UK’s size. As most will agree, inspecting the structural health of the 17,439 bridges in our portfolio is more than a two person job and costs billions each year in inspection and maintenance cost.
So if we could determine a bridge’s condition based on the condition of similar bridges we would be able to identify issues more quickly and in a more targeted way than sending a team around the state evaluating each bridge’s condition. The AI approach allows us to identify systemic issues – for example, damages due to weather conditions, higher than expected usage or asset manager/subcontractor faults.
All bridges in our portfolio are inspected and receive a rating from 1 to 7, with 7 being in new condition and a rating of 5 or greater considered as good condition. We can see below that the ratings for old bridges have greater variation than the new bridges and that the rating of new bridges is closely correlated with the year it was built.
AI Driven Decisions in Construction Asset Management
Here we are using an unsupervised learning algorithm which classifies bridges based on a sample of known bridge ratings. In other words, we use previous bridge inspection data to predict what condition a bridge is in that we have not yet inspected. As there seems to be a difference in trend between new bridges and old bridges, we built a model for each group to get our estimates as close as possible to the actual rating.
In order to implement the algorithm we only use 7 variables such as Built Year, Owner and several location variables (i.e. Region, County, Municipality). We have not taken into account weather, usage or other operational data.
Without knowing anything about the portfolio, we are able to generate important insights.
- The Region is the most important predictor for a bridges rating, which is interesting. This could be to do with the fact that some regions have a higher proportion of bridges, but never the less we would have expected the built year to be more important.
- The Municipality is the third most important predictor, which could mean that some geographic regions are better at maintaining bridges than others or… that inspectors/engineers in some regions are more likely give bridges better ratings than others.
- The Owner of the bridge is less important when comes to determining the rating which is surprising assuming that the owner is likely the governance body allocating funds to keep up the network for the bridges it owns.
Because we are interested in how to manage the entire portfolio of bridges more effectively, we implemented the model and visualised the results in Google Data Studio.
Before we wrap up, let’s look at how well we are actually able to predict these ratings. For new bridges, we were able to predict results to 92% accurately. This means, if you use an AI-driven approach, your rating might be off by 0.47 on the rating scale. For old bridges with greater variation in conditions, we were able to predict the rating to 88% accurately – or off by 0.62 on the rating scale.
Making insights actionable
Knowing what we know now about the portfolio and the model, how can you implement this to streamline your operation? It’s important to understand that “AI Driven” does not mean blind faith – inspections are important and experts should perform rigorous inspections to evaluate a structure’s condition and health. We advise our clients to use a machine guided approach to maximise the value structural engineers create:
- All assets in the portfolio with an unsatisfactory rating should be examined and a plan put in place to address critical issues
- Teams will be organised to inspect a sample of bridges thoroughly which will be fed back into the model continuously to estimate the condition of all bridges in the portfolio
- Warning analytics will be implemented that alert teams when problem trends are spotted
- Team sizes can be reduced and re-purposed to focus on implementing fixes rather than inspection
- Further enhancements can be made through the addition of operational data and the implementation of sensors directly connected to the model to provide real-time insights into bridge conditions and risks.
We estimate that the time spent on inspections could be reduced by 40-50% after successful implementation, with a 30-40% increase in productivity.
If you would like to learn more about how AI Driven Construction Asset Management can be implemented contact us today.